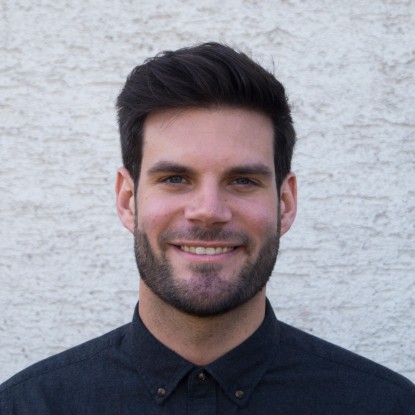
Matthias Schultheis M.Sc.
Psychologie der Informationsverarbeitung
Kontakt
matthias.schultheis@tu-...
work +49 6151 16-57 423
Work
S1|15 235
Alexanderstraße 10
64283
Darmstadt
Matthias Schultheis joined the lab in December 2019 as a PhD student. He works on explainable models for human and machine intelligence within the and is jointly supervised by Whitebox project and Prof. Rothkopf. Matthias is also a member of the Prof. Koeppl of TU Darmstadt. Self-Organizing Systems (SOS) Lab
Research Interests
His research aims at understanding and predicting human and machine behavior using methods for Inverse Reinforcement Learning. To this end, he works on methodology that characterizes preferences of human subjects and autonomous agents based on sequential decision data. In general, he is interested in various topics related to Reinforcement Learning, Preference Elicitation, and Bayesian Modelling.
Bio
Before starting his PhD, he completed his Bachelor's degree in Computer Science and Master's degree in Autonomous Systems at the Technische Universität Darmstadt. During his studies, he spent some time at ENSEEIHT in Toulouse (France) and at Universidad Nacional de Córdoba (Argentina). In his Master's thesis, entitled Approximate Bayesian Reinforcement Learning for System Identification, he investigated model-based solutions for directed exploration in learning systems. In his Bachelor's studies he was a member of the Athena-Minerva Cybathlon-Team of TU Darmstadt and the Max Planck Institute for Intelligent Systems in Tübingen, which developed a Brain-Computer-Interface (BCI) system and participated in the BCI Race at the Cybathlon 2016 in Zürich (Switzerland).
Peer-Reviewed Publications
- Straub, D.*, Schultheis, M.*, Koeppl, H., & Rothkopf, C. A. (2023) Probabilistic inverse optimal control for non-linear partially observable systems disentangles perceptual uncertainty and behavioral costs. In Advances in Neural Information Processing Systems (NeurIPS), (accepted)
[] [ preprint] code - Schultheis, M., Rothkopf, C. A., & Koeppl, H. (2022). Reinforcement learning with non-exponential discounting. In Advances in Neural Information Processing Systems (NeurIPS), 35:3649-3662.
[ (wird in neuem Tab geöffnet)] [ paper] [ code] talk - Schultheis, M.*, Straub, D.*, & Rothkopf, C. A. (2021). Inverse optimal control adapted to the noise characteristics of the human sensorimotor system. In Advances in Neural Information Processing Systems (NeurIPS), 34:9429-9442.
[ (wird in neuem Tab geöffnet)] [ paper] [ code] talk - Alt, B., Schultheis, M., & Koeppl, H. (2020). POMDPs in continuous time and discrete spaces. In Advances in Neural Information Processing Systems (NeurIPS), 33:13151-13162.
[ (wird in neuem Tab geöffnet)] [ paper] code - Schultheis, M., Belousov, B., Abdulsamad, H., & Peters, J. (2020). Receding horizon curiosity. In Proceedings of the Conference on Robot Learning (CoRL), 100:1278-1288.
[ (wird in neuem Tab geöffnet)] [ paper] code